Simple fuzzy echo classification from dual-pol moments#
[1]:
import wradlib
from wradlib.util import get_wradlib_data_file
import os
import numpy as np
import matplotlib.pyplot as plt
import warnings
import xarray as xr
warnings.filterwarnings("ignore")
try:
get_ipython().run_line_magic("matplotlib inline")
except:
plt.ion()
/home/runner/micromamba/envs/wradlib-tests/lib/python3.11/site-packages/h5py/__init__.py:36: UserWarning: h5py is running against HDF5 1.14.3 when it was built against 1.14.2, this may cause problems
_warn(("h5py is running against HDF5 {0} when it was built against {1}, "
Setting the file paths#
[2]:
rhofile = get_wradlib_data_file("netcdf/TAG-20120801-140046-02-R.nc")
phifile = get_wradlib_data_file("netcdf/TAG-20120801-140046-02-P.nc")
reffile = get_wradlib_data_file("netcdf/TAG-20120801-140046-02-Z.nc")
dopfile = get_wradlib_data_file("netcdf/TAG-20120801-140046-02-V.nc")
zdrfile = get_wradlib_data_file("netcdf/TAG-20120801-140046-02-D.nc")
mapfile = get_wradlib_data_file("hdf5/TAG_cmap_sweeps_0204050607.hdf5")
Downloading file 'netcdf/TAG-20120801-140046-02-R.nc' from 'https://github.com/wradlib/wradlib-data/raw/pooch/data/netcdf/TAG-20120801-140046-02-R.nc' to '/home/runner/work/wradlib/wradlib/wradlib-data'.
Downloading file 'netcdf/TAG-20120801-140046-02-P.nc' from 'https://github.com/wradlib/wradlib-data/raw/pooch/data/netcdf/TAG-20120801-140046-02-P.nc' to '/home/runner/work/wradlib/wradlib/wradlib-data'.
Downloading file 'netcdf/TAG-20120801-140046-02-Z.nc' from 'https://github.com/wradlib/wradlib-data/raw/pooch/data/netcdf/TAG-20120801-140046-02-Z.nc' to '/home/runner/work/wradlib/wradlib/wradlib-data'.
Downloading file 'netcdf/TAG-20120801-140046-02-V.nc' from 'https://github.com/wradlib/wradlib-data/raw/pooch/data/netcdf/TAG-20120801-140046-02-V.nc' to '/home/runner/work/wradlib/wradlib/wradlib-data'.
Downloading file 'netcdf/TAG-20120801-140046-02-D.nc' from 'https://github.com/wradlib/wradlib-data/raw/pooch/data/netcdf/TAG-20120801-140046-02-D.nc' to '/home/runner/work/wradlib/wradlib/wradlib-data'.
Downloading file 'hdf5/TAG_cmap_sweeps_0204050607.hdf5' from 'https://github.com/wradlib/wradlib-data/raw/pooch/data/hdf5/TAG_cmap_sweeps_0204050607.hdf5' to '/home/runner/work/wradlib/wradlib/wradlib-data'.
Read the data (radar moments and static clutter map)#
[3]:
# We need to organize our data as a dictionary
dat = {}
dat["rho"], attrs_rho = wradlib.io.read_edge_netcdf(rhofile)
dat["phi"], attrs_phi = wradlib.io.read_edge_netcdf(phifile)
dat["ref"], attrs_ref = wradlib.io.read_edge_netcdf(reffile)
dat["dop"], attrs_dop = wradlib.io.read_edge_netcdf(dopfile)
dat["zdr"], attrs_zdr = wradlib.io.read_edge_netcdf(zdrfile)
dat["map"] = wradlib.io.from_hdf5(mapfile)[0][0]
dat = {k: (["azimuth", "range"], v) for k, v in dat.items()}
[4]:
az, rng = dat["rho"][1].shape
swp = xr.Dataset(dat, coords={"azimuth": np.arange(az), "range": np.arange(rng)})
swp = swp.assign_coords(
dict(
longitude=7,
latitude=53,
altitude=0,
elevation=1,
sweep_mode="azimuth_surveillance",
)
)
swp = swp.wrl.georef.georeference()
display(swp)
<xarray.Dataset> Dimensions: (azimuth: 360, range: 240) Coordinates: (12/14) * azimuth (azimuth) int64 0 1 2 3 4 5 6 7 ... 353 354 355 356 357 358 359 * range (range) int64 0 1 2 3 4 5 6 7 ... 233 234 235 236 237 238 239 longitude int64 7 latitude int64 53 altitude int64 0 elevation int64 1 ... ... y (azimuth, range) float64 0.0 0.9998 2.0 ... 236.9 237.9 238.9 z (azimuth, range) float64 0.0 0.01745 0.03491 ... 4.157 4.174 gr (azimuth, range) float64 0.0 0.9998 2.0 ... 237.0 238.0 239.0 rays (azimuth, range) int64 0 0 0 0 0 0 0 ... 359 359 359 359 359 359 bins (azimuth, range) int64 0 1 2 3 4 5 6 ... 234 235 236 237 238 239 crs_wkt int64 0 Data variables: rho (azimuth, range) float32 0.8743 0.751 0.8156 ... 0.2174 0.2788 phi (azimuth, range) float32 50.12 50.12 50.12 50.12 ... nan nan nan ref (azimuth, range) float32 -15.5 -18.5 -12.0 -7.0 ... nan nan nan dop (azimuth, range) float32 -0.4297 -0.4297 -0.4297 ... nan nan nan zdr (azimuth, range) float32 8.0 -7.059 -0.1882 ... nan nan nan map (azimuth, range) bool True True True True ... False False False
Identify non-meteorological echoes using fuzzy echo classification#
See Crisologo et al. (2015) and Vulpiani et al. (2012) for details.
[5]:
moments = dict(rho="rho", phi="phi", ref="ref", dop="dop", zdr="zdr", map="map")
weights = {"zdr": 0.4, "rho": 0.4, "rho2": 0.4, "phi": 0.1, "dop": 0.1, "map": 0.5}
prob, nanmask = swp.wrl.classify.classify_echo_fuzzy(moments, weights=weights)
thresh = 0.5
cmap = prob.where(prob < thresh, True, False)
View classfication results#
[6]:
fig = plt.figure(figsize=(12, 5))
# Horizontal reflectivity
ax = plt.subplot(121, aspect="equal")
pm = swp.ref.plot(x="x", y="y", ax=ax, cbar_kwargs=dict(label="dBZ"))
ax = wradlib.vis.plot_ppi_crosshair(site=(0, 0, 0), ranges=[80, 160, 240])
plt.xlim(-240, 240)
plt.ylim(-240, 240)
plt.xlabel("# bins from radar")
plt.ylabel("# bins from radar")
# Echo classification
ax = plt.subplot(122, aspect="equal")
pm = cmap.where(~np.isnan(swp.ref)).plot(
x="x",
y="y",
ax=ax,
cmap="bwr",
cbar_kwargs=dict(label="meterol. echo=0 - non-meteorol. echo=1"),
)
ax = wradlib.vis.plot_ppi_crosshair(site=(0, 0, 0), ranges=[80, 160, 240])
plt.xlim(-240, 240)
plt.ylim(-240, 240)
plt.xlabel("# bins from radar")
plt.ylabel("# bins from radar")
[6]:
Text(0, 0.5, '# bins from radar')
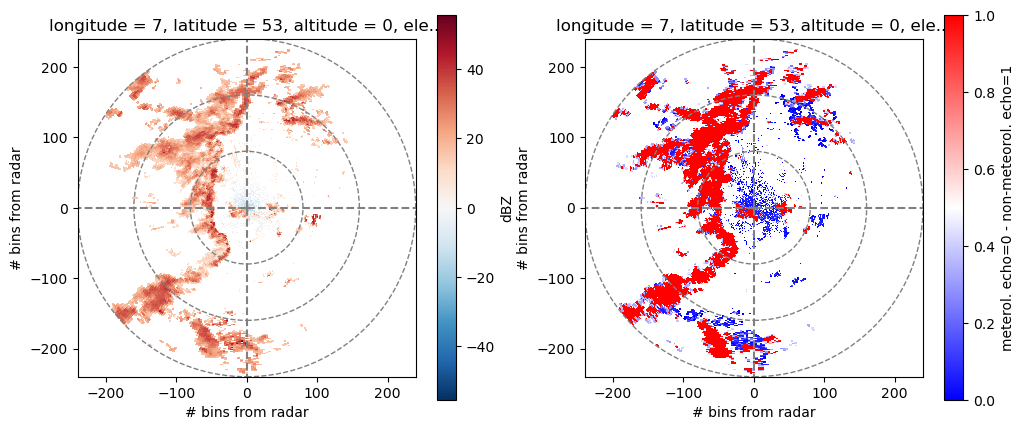